CrowdMic:
Enabling CrowdSourced Recordings
The CrowdMic Project
There is no doubt that we live in an environment that is massively recorded by multiple people at any point in time. Although we have the technology to combine such information in the visual space (e.g. with PhotoSynth), there is no good way to combine audio streams from multiple recordings of the same event. This projects fills that gap by utilizing new advances in spectral decompositions and landmark methods to help us take large amounts of audio recordings of the same event and resynthesize them as one high-quality version, eliminating the artifacts and noise of each individual recording while taking advantage of their strong points.
This project aims to introduce new computational tools to combine uncurated recordings at a large scale, and will allow us to glean information that no single recording can provide. By combining all available information and producing objective representations we can easily sift through data from massively recorded events (e.g. social unrest, historical moments) and focus on the needed information. This will also allow us to produce high-quality recordings from historical events that might not otherwise be well documented, by using the power of the crowds. Results will be distributed at this web site, and will be incorporated in the development of classes on social and crowdsourcing aspects of audio and signal processing. A service that allows the consolidation from user-submitted recordings will be setup, and the methods will be distributed in both publication and source code form to stimulate activity in this field.
You can try out a beta version here.
Resulting Publications and Presentations:
1. Paris Smaragdis and Minje Kim (2013), "Non-Negative Matrix Factorization for Irregularly-Spaced Transforms," in Proceedings of the IEEE Workshop on Applications of Signal Processing to Audio and Acoustics (WASPAA), New Paltz, NY.
2. Minje Kim and Paris Smaragdis (2013), "Manifold Preserving Hierarchical Topic Models for Quantization and Approximation," in
Proceedings of the International Conference on Machine Learning (ICML), Atlanta, GA.
3. Minje Kim and Paris Smaragdis (2013), “Collaborative Audio Enhancement Using Probabilistic Latent Component Sharing,” in Proceedings of the IEEE International Conference on Acoustics, Speech, and Signal Processing (ICASSP), Vancouver, BC, Canada. [Demo]. Winner of the Google ICASSP Student Travel Grants, Best Student Paper Award finalist.
4. Sübakan, Y.C., J. Traa and P. Smaragdis. 2014. Spectral Learning of Mixture of Hidden Markov Models, in Neural Information Processing Systems (NIPS) 2014. Montreal, Canada.
5. Minje Kim and Paris Smaragdis, "Collaborative Audio Enhancement: Crowdsourced Audio Recording," Neural Information Processing Systems (NIPS) Workshop on Crowdsourcing and Machine Learning, Montreal, Canada, Dec. 8-13, 2014.
6. Kim, M. and P. Smaragdis. 2014. Efficient Model Selection for Speech Enhancement Using a Deflation Method for Nonnegative Matrix Factorization, in IEEE GlobalSIP 2014, Atlanta, Georgia.
7. Kim, M., P. Smaragdis, and G.J. Mysore. 2015, Efficient Manifold Preserving Audio Source Separation Using Locality Sensitive Hashing, IEEE International Conference on Acoustics, Speech, and Signal Processing (ICASSP), Brisbane, Australia, April 19-24, 2015
8. Kim, M. and P. Smaragdis. 2016. Efficient Neighborhood-Based Topic Modeling for Collaborative Audio Enhancement on Massive Crowdsourced Recordings, IEEE International Conference on Acoustics, Speech and Signal Processing (ICASSP), Shanghai, China, March 20-25, 2016.
9. Correa Carvalho, R.G., and P. Smaragdis. 2017. Towards End-to-end Polyphonic Music Transcription: Transforming Music Audio Directly to a Score, in IEEE Workshop for Applications of Signal Processing to Audio and Acoustics (WASPAA), New Paltz, NY, USA. October 2017.
10. Casebeer, J., B. Luc, and P. Smaragdis. Multi-View Networks for Denoising of Arbitrary Numbers of Channels, in IEEE International Workshop on Acoustic Signal Enhancement, September, Tokyo, Japan.
11. Keynote presentation, in IEEE 19th International Workshop on Multimedia Signal Processing. Bedfordshire, UK, October 2017.
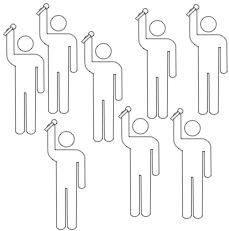
This material is based upon work supported by the National Science Foundation under Grant: III: Small: MicSynth: Enhancing and Reconstructing Sound Scenes from Crowdsourced Recordings. Award #:1319708
Any opinions, findings, and conclusions or recommendations expressed in this material are those of the author(s) and do not necessarily reflect the views of the National Science Foundation.
Thi page was last updated on Jul 26, 2018